What DSPs Does the Cloud Edge Need?
By storing and processing data closer to the end user and reducing latency, smaller data centers on the network edge significantly impact how networks are designed and implemented. These benefits are causing the global market for edge data centers to explode, with PWC predicting that it will nearly triple from $4 billion in 2017 to $13.5 billion in 2024. Various trends are driving the rise of the edge cloud: 5G networks and the Internet of Things (IoT), augmented and virtual reality applications, network function virtualization, and content delivery networks.
Several of these applications require lower latencies than before, and centralized cloud computing cannot deliver those data packets quickly enough. As shown in Table 1, a data center on a town or suburb aggregation point could halve the latency compared to a centralized hyperscale data center. Enterprises with their own data center on-premises can reduce latencies by 12 to 30 times compared to hyperscale data centers.
Type of Edge | Datacenter | Location | Number of DCs per 10M people | Average Latency | Size | |
On-premises edge | Enterprise site | Businesses | NA | 2-5 ms | 1 rack max | |
Network (Mobile) | Tower edge | Tower | Nationwide | 3000 | 10 ms | 2 racks max |
Outer edge | Aggregation points | Town | 150 | 30 ms | 2-6 racks | |
Inner edge | Core | Major city | 10 | 40 ms | 10+ racks | |
Regional edge | Regional | Major city | 100 | 50 ms | 100+ racks | |
Not edge | Hyperscale | State/national | 1 | 60+ ms | 5000+ racks |
This situation leads to hyperscale data center providers cooperating with telecom operators to install their servers in the existing carrier infrastructure. For example, Amazon Web Services (AWS) is implementing edge technology in carrier networks and company premises (e.g., AWS Wavelength, AWS Outposts). Google and Microsoft have strategies and products that are very similar. In this context, edge computing poses a few problems for telecom providers. They must manage hundreds or thousands of new nodes that will be hard to control and maintain.
These conditions mean that optical transceivers for these networks, and thus their digital signal processors (DSPs), must have flexible and low power consumption and smart features that allow them to adapt to different network conditions.
Using Adaptable Power Settings
Reducing power consumption in the cloud edge is not just about reducing the maximum power consumption of transceivers. Transceivers and DSPs must also be smart and decide whether to operate on low- or high-power mode depending on the optical link budget and fiber length. For example, if the transceiver must operate at its maximum capacity, a programmable interface can be controlled remotely to set the amplifiers at maximum power. However, if the operator uses the transceiver for just half of the maximum capacity, the transceiver can operate with lower power on the amplifiers. The transceiver uses energy more efficiently and sustainably by adapting to these circumstances.
Fiber monitoring is also an essential variable in this equation. A smart DSP could change its modulation scheme or lower the power of its semiconductor optical amplifier (SOA) if telemetry data indicates a good quality fiber. Conversely, if the fiber quality is poor, the transceiver can transmit with a more limited modulation scheme or higher power to reduce bit errors. If the smart pluggable detects that the fiber length is relatively short, the laser transmitter power or the DSP power consumption could be scaled down to save energy.
The Importance of a Co-Design Philosophy for DSPs
Transceiver developers often source their DSP, laser, and optical engine from different suppliers, so all these chips are designed separately. This setup reduces the time to market and simplifies the research and design processes, but it comes with performance and power consumption trade-offs.
In such cases, the DSP is like a Swiss army knife: a jack of all trades designed for different kinds of PIC but a master of none. Given the ever-increasing demand for capacity and the need for sustainability both as financial and social responsibility, transceiver developers increasingly need a steak knife rather than a Swiss army knife.
As we explained in a previous article about fit-for-platform DSPs, a transceiver optical engine designed on the indium phosphide platform could be designed to run at a voltage compatible with the DSP’s signal output. This way, the optimized DSP could drive the PIC directly without needing a separate analog driver, doing away with a significant power conversion overhead compared to a silicon photonics setup, as shown in the figure below.
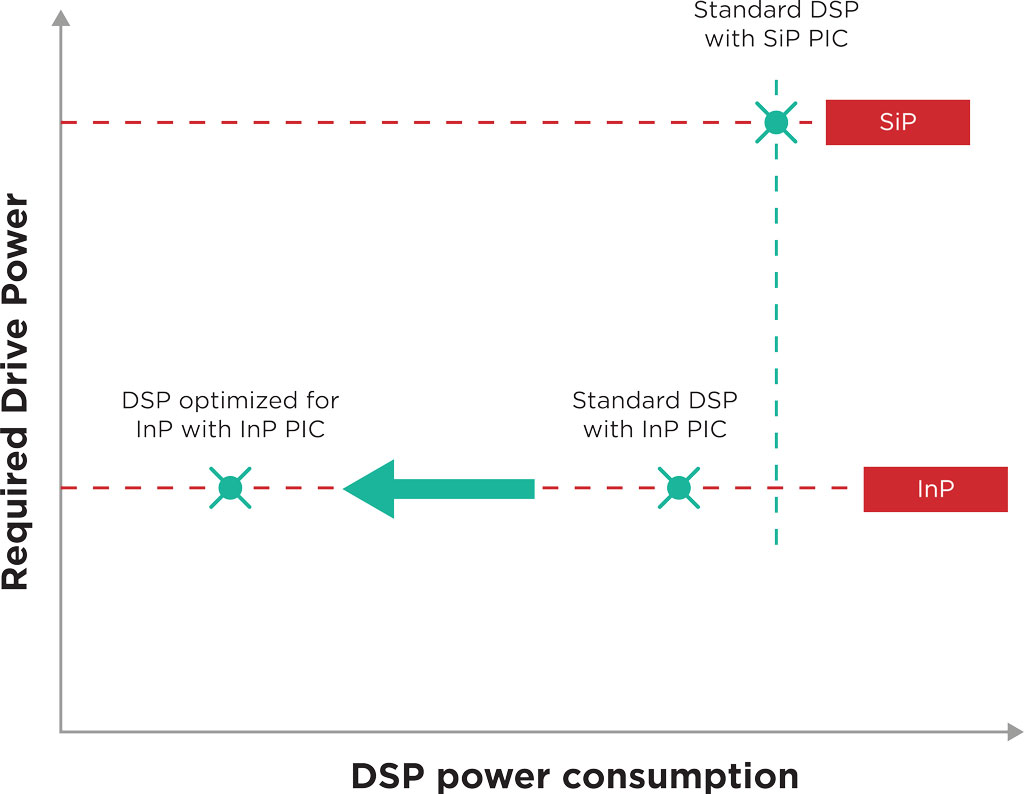
Scaling the Edge Cloud with Automation
With the rise of edge data centers, telecom providers must manage hundreds or thousands of new nodes that will take much work to control and maintain. Furthermore, providers also need a flexible network with pay-as-you-go scalability that can handle future capacity needs. Automation is vital to achieving such flexibility and scalability.
Automation potential improves further by combining artificial intelligence with the software-defined networks (SDNs) framework that virtualizes and centralizes network functions. This creates an automated and centralized management layer that can allocate resources efficiently and dynamically. For example, the AI network controller can take telemetry data from the whole network to decide where to route traffic and adjust power levels, reducing power consumption.
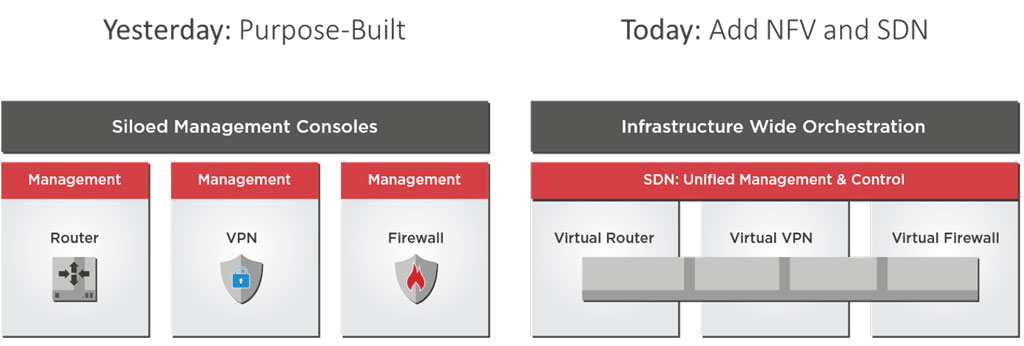
In this context, smart digital signal processors (DSPs) and transceivers can give the AI controller more degrees of freedom to optimize the network. They could provide more telemetry to the AI controller so that it makes better decisions. The AI management layer can then remotely control programmable interfaces in the transceiver and DSP so that the optical links can adjust the varying network conditions. If you want to know more about these topics, you can read last week’s article about transceivers in the age of AI.
Takeaways
Cloud-native applications require edge data centers that handle increased traffic and lower network latency. However, their implementation came with the challenges of more data center interconnects and a massive increase in nodes to manage. Scaling edge data center networks will require greater automation and more flexible power management, and smarter DSPs and transceivers will be vital to enable these goals.
Co-design approaches can optimize the interfacing of the DSP with the optical engine, making the transceiver more power efficient. Further power consumption gains can also be achieved with smarter DSPs and transceivers that provide telemetry data to centralized AI controllers. These smart network components can then adjust their power output based on the decisions and instructions of the AI controller.