Artificial intelligence (AI) will have a significant role in making optical networks more scalable, affordable,…
Artificial intelligence (AI) will have a significant role in making optical networks more scalable, affordable, and sustainable. It can gather information from devices across the optical network to identify patterns and make decisions independently without human input. By synergizing with other technologies, such as network function virtualization (NFV), AI can become a centralized management and orchestration network layer. Such a setup can fully automate network provisioning, diagnostics, and management, as shown in the diagram below.
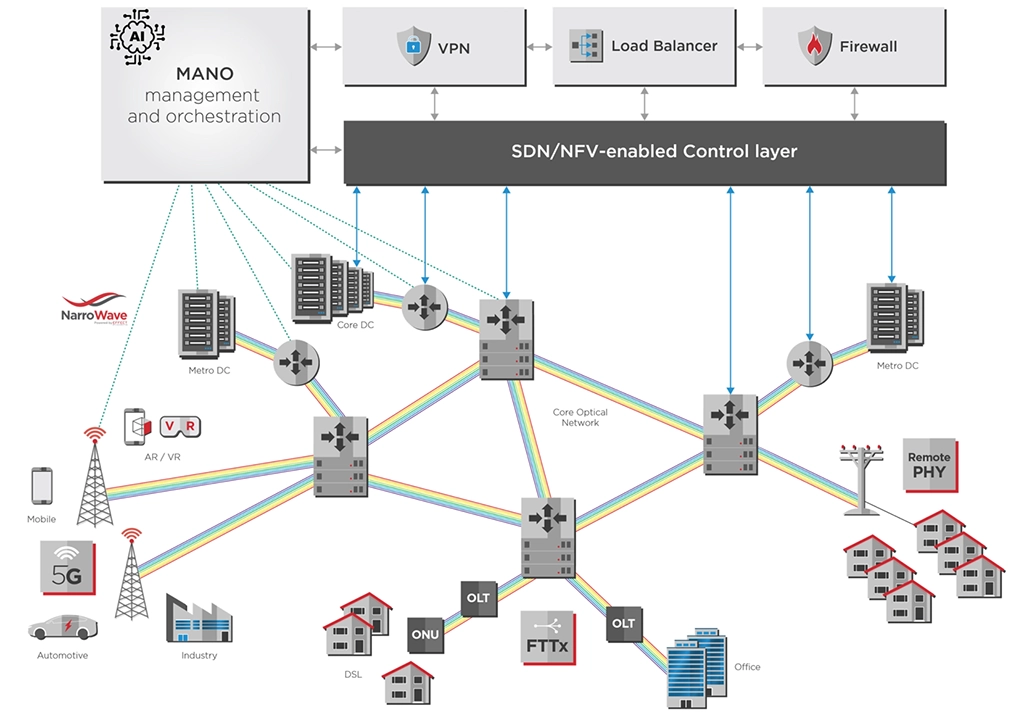
However, artificial intelligence and machine learning algorithms are data-hungry. To work optimally, they need information from all network layers and ever-faster data centers to process it quickly. Pluggable optical transceivers thus need to become smarter, relaying more information back to the AI central unit, and faster, enabling increased AI processing.
The Need for Faster Transceivers
Optical transceivers are crucial in developing better AI systems by facilitating the rapid, reliable data transmission these systems need to do their jobs. High-speed, high-bandwidth connections are essential to interconnect data centers and supercomputers that host AI systems and allow them to analyze a massive volume of data.
In addition, optical transceivers are essential for facilitating the development of artificial intelligence-based edge computing, which entails relocating compute resources to the network’s periphery. This is essential for facilitating the quick processing of data from Internet-of-Things (IoT) devices like sensors and cameras, which helps minimize latency and increase reaction times.
400 Gbps links are becoming the standard across data center interconnects, but providers are already considering the next steps. LightCounting forecasts significant growth in the shipments of dense-wavelength division multiplexing (DWDM) ports with data rates of 600G, 800G, and beyond in the next five years. We discuss these solutions in greater detail in our article about the roadmap to 800G and beyond.
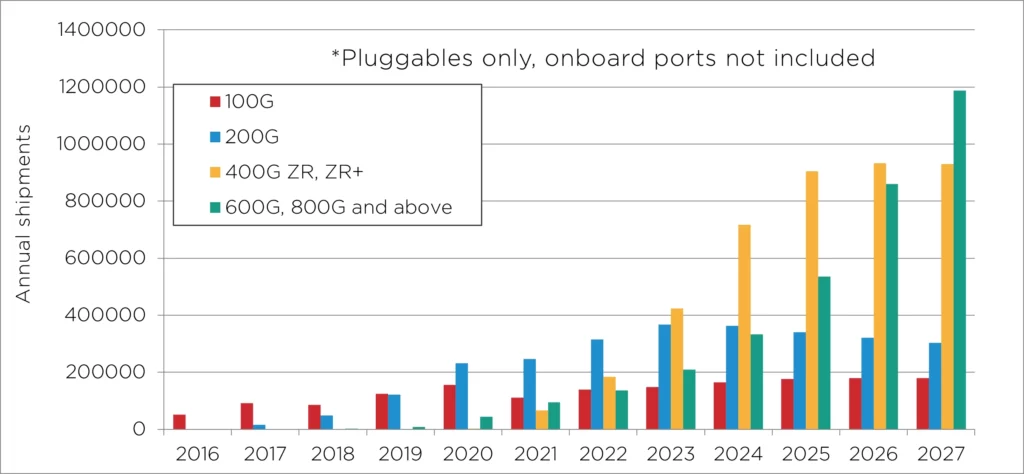
The Need for Telemetry Data
Mobile networks now and in the future will consist of a massive number of devices, software applications, and technologies. Self-managed, zero-touch automated networks will be required to handle all these new devices and use cases. Realizing this full network automation requires two vital components.
- Artificial intelligence and machine learning algorithms for comprehensive network automation: For instance, AI in network management can drastically cut the energy usage of future telecom networks.
- Sensor and control data flow across all network model layers, including the physical layer: As networks grow in size and complexity, the management and orchestration (MANO) software needs more degrees of freedom and dials to turn.
These goals require smart optical equipment and components that provide comprehensive telemetry data about their status and the fiber they are connected to. The AI-controlled centralized management and orchestration layer can then use this data for remote management and diagnostics. We discuss this topic further in our previous article on remote provisioning, diagnostics, and management.
For example, a smart optical transceiver that fits this centralized AI-management model should relay data to the AI controller about fiber conditions. Such monitoring is not just limited to finding major faults or cuts in the fiber but also smaller degradations or delays in the fiber that stem from age, increased stress in the link due to increased traffic, and nonlinear optical effects. A transceiver that could relay all this data allows the AI controller to make better decisions about how to route traffic through the network.
A Smart Transceiver to Rule All Network Links
After relaying data to the AI management system, a smart pluggable transceiver must also switch parameters to adapt to different use cases and instructions given by the controller.
Let’s look at an example of forward error correction (FEC). FEC makes the coherent link much more tolerant to noise than a direct detect system and enables much longer reach and higher capacity. In other words, FEC algorithms allow the DSP to enhance the link performance without changing the hardware. This enhancement is analogous to imaging cameras: image processing algorithms allow the lenses inside your phone camera to produce a higher-quality image.
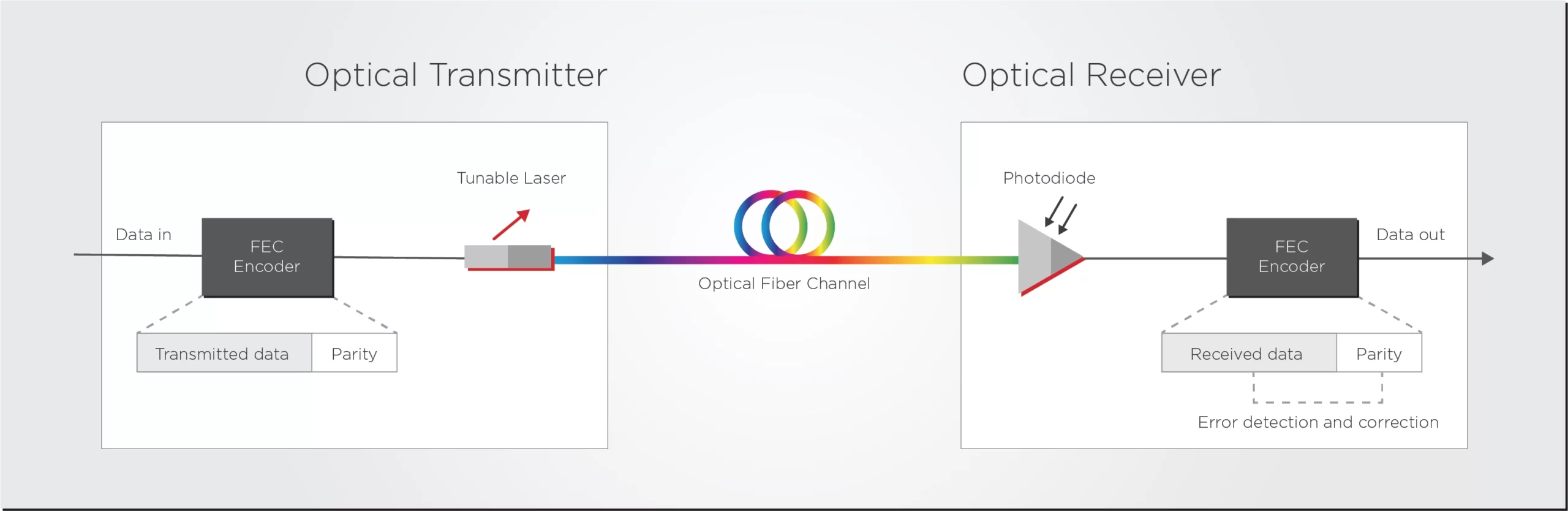
A smart transceiver and DSP could switch among different FEC algorithms to adapt to network performance and use cases. Let’s look at the case of upgrading a long metro link of 650km running at 100 Gbps with open FEC. The operator needs to increase that link capacity to 400 Gbps, but open FEC could struggle to provide the necessary link performance. However, if the transceiver can be remotely reconfigured to use a proprietary FEC standard, the transceiver will be able to handle this upgraded link.
Reconfigurable transceivers can also be beneficial to auto-configure links to deal with specific network conditions, especially in brownfield links. Let’s return to the fiber monitoring subject we discussed in the previous section. A transceiver can change its modulation scheme or lower the power of its semiconductor optical amplifier (SOA) if telemetry data indicates a good quality fiber. Conversely, if the fiber quality is poor, the transceiver can transmit with a more limited modulation scheme or higher power to reduce bit errors. If the smart pluggable detects that the fiber length is relatively short, the laser transmitter power or the DSP power consumption could be scaled down to save energy.
Takeaways
Optical networks will need artificial intelligence and machine learning to scale more efficiently and affordably to handle the increased traffic and connected devices. Conversely, AI systems will also need faster pluggables than before to acquire data and make decisions more quickly. Pluggables that fit this new AI era must be fast, smart, and adapt to multiple use cases and conditions. They will need to scale up to speeds beyond 400G and relay monitoring data back to the AI management layer in the central office. The AI management layer can then program transceiver interfaces from this telemetry data to change parameters and optimize the network.
Tags: 400 Gbps links, affordable networks, AI networks, centralized management, data-hungry algorithms, Dense wavelength division multiplexing, digital signal processing, EFFECT Photonics, Fiber Monitoring, forward error correction, high-speed transceivers, mobile networks, Network automation, Network Function Virtualization, network orchestration, network scalability, optical pluggables, Reconfigurable transceivers, smart optical equipment, sustainable networks, telemetry data