How Photonics Enables AI Networks
Artificial Intelligence (AI) networks have revolutionized various industries by enabling tasks such as image recognition, natural language processing, and autonomous driving. Central to the functioning of AI networks are two processes: AI training and AI inference. AI training involves feeding large datasets into algorithms to learn patterns and make predictions, typically requiring significant computational resources. AI inference, on the other hand, is the process of using trained models to make predictions on new data, which requires efficient and fast computation. As the demand for AI capabilities grows, the need for robust, high-speed, and energy-efficient interconnects within data centers and between network nodes becomes critical. This is where photonics comes into play, offering significant advantages over traditional electronic methods.
Enhancing Data Center Interconnects
Data centers are the backbone of AI networks, housing the vast computational resources needed for both training and inference tasks. As AI models become more complex, the data traffic within and between data centers increases exponentially. Traditional electronic interconnects face limitations in terms of bandwidth and power efficiency. Photonics, using light to transmit data, offers a solution to these challenges.
Photonics enables the integration of optical components like lasers, modulators, and detectors on a single chip. This technology allows for high-speed data transfer with significantly lower power consumption compared to electronic interconnects. These advancements are crucial for handling the data-intensive nature of AI workloads.
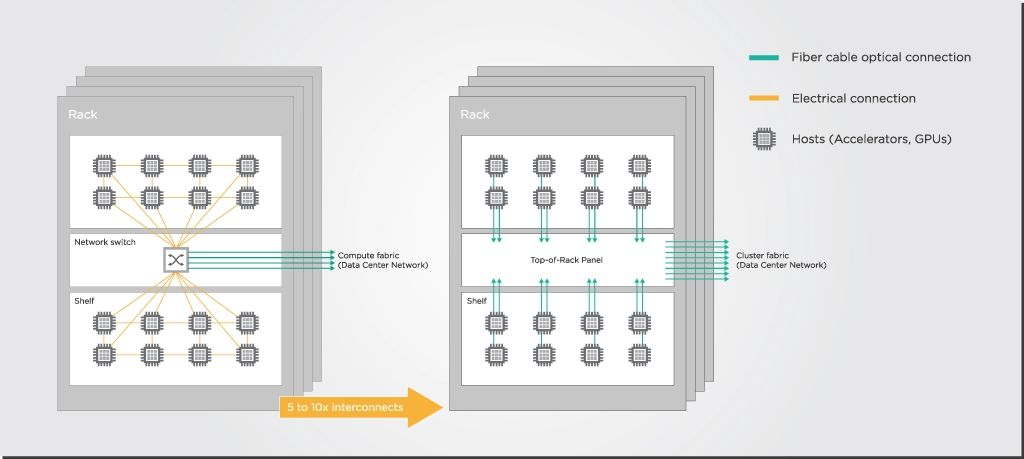
Enabling High-Speed AI Training and Inference
AI training requires the processing of vast amounts of data, often necessitating the use of distributed computing resources across multiple data centers. Photonic interconnects facilitate this by providing ultra-high bandwidth connections, which are essential for the rapid movement of data between computational nodes. The high-speed data transfer capabilities of photonics reduce latency and improve the overall efficiency of AI training processes.
This high transfer speed and capacity also plays a critical role in AI inference, particularly in scenarios where real-time processing and high throughput is essential. For example, in a network featuring autonomous vehicles, AI inference must process data from sensors and cameras in real-time to make immediate decisions. For other ways in which photonics plays a role in autonomous vehicles, please read our article on LIDAR and photonics.
Into Network Edge Applications
The network edge refers to the point where data is generated and collected, such as IoT devices, sensors, and local servers. Deploying AI capabilities at the network edge allows for real-time data processing and decision-making, reducing the need to send data back to centralized data centers. This approach not only reduces latency but also enhances data privacy and security by keeping sensitive information local.
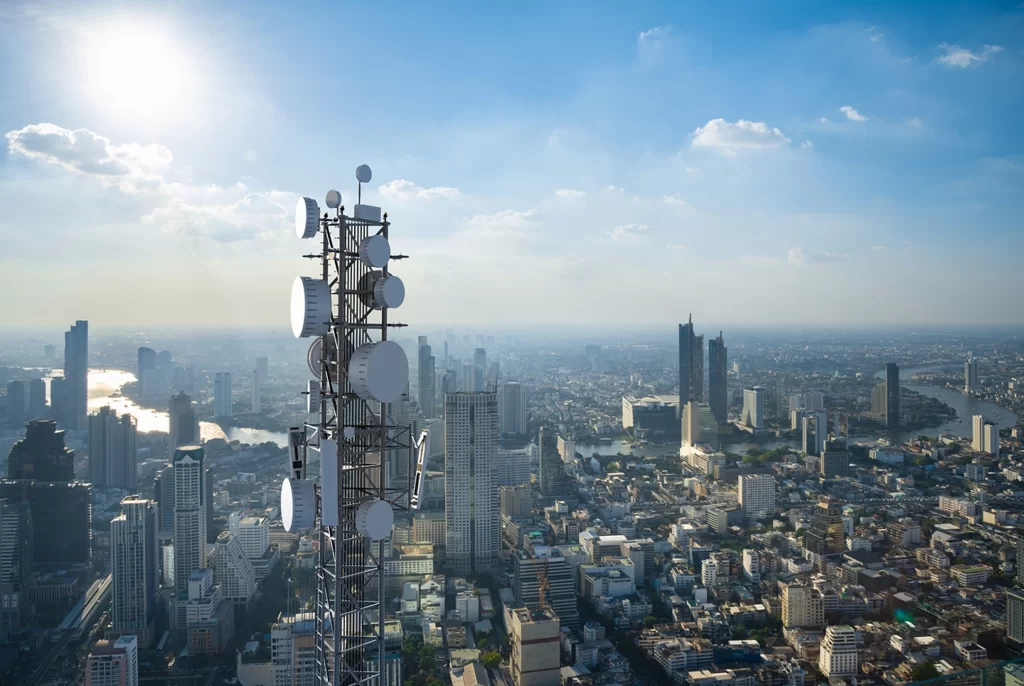
Photonics enables edge AI by providing the necessary high-speed, low-power interconnects required for efficient data processing at the edge. For some use cases, the network edge could benefit from upgrading their existing direct detect or grey links to 100G DWDM coherent. However, the industry needs more affordable and power-efficient transceivers and DSPs specifically designed for coherent 100G transmission in edge and access networks. By realizing DSPs co-designed with the optics, adjusted for reduced power consumption, and industrially hardened, the network edge will have coherent DSP and transceiver products adapted to their needs. This is a path EFFECT Photonics believes strongly in, and we talk more about it in one of our previous articles.
Conclusion
Photonics is transforming the landscape of AI networks by providing high-speed, energy-efficient interconnects that enhance data center performance, enable faster AI training and inference, and support real-time processing at the network edge. As AI continues to evolve and expand into new applications, the role of photonics will become increasingly critical in addressing the challenges of bandwidth, latency, and power consumption. By leveraging the unique properties of light, photonics offers a path to more efficient and scalable AI networks, driving innovation and enabling new possibilities across various industries.