AI at the Network Edge
Artificial Intelligence (AI) can impact several different industries by enhancing efficiency, automation, and data processing capabilities. The network edge is another area where AI can deliver such improvements. Edge computing, combined with AI, enables data processing closer to the source of data generation, leading to reduced latency, improved real-time data analytics, and enhanced security. This article delves into the potential of AI at the network edge, exploring its applications, training and inference processes, and future impact.
The Potential of AI at the Network Edge
According to market research, the global market for edge computing technologies is projected to grow from $46.3 billion in 2022 to $124.7 billion by 2027.
AI at the network edge involves deploying AI models and algorithms closer to where data is generated, such as in IoT devices, sensors, and local servers. This proximity allows for real-time data processing and decision-making, which is critical for applications that require immediate responses. Industries such as manufacturing, healthcare, retail, and smart cities are prime beneficiaries of edge AI. For instance, in manufacturing, edge AI can monitor machinery in real-time to predict and prevent failures, enhancing operational efficiency and reducing downtime. In healthcare, edge AI enables real-time patient monitoring, providing immediate alerts to medical staff about critical changes in patient conditions.
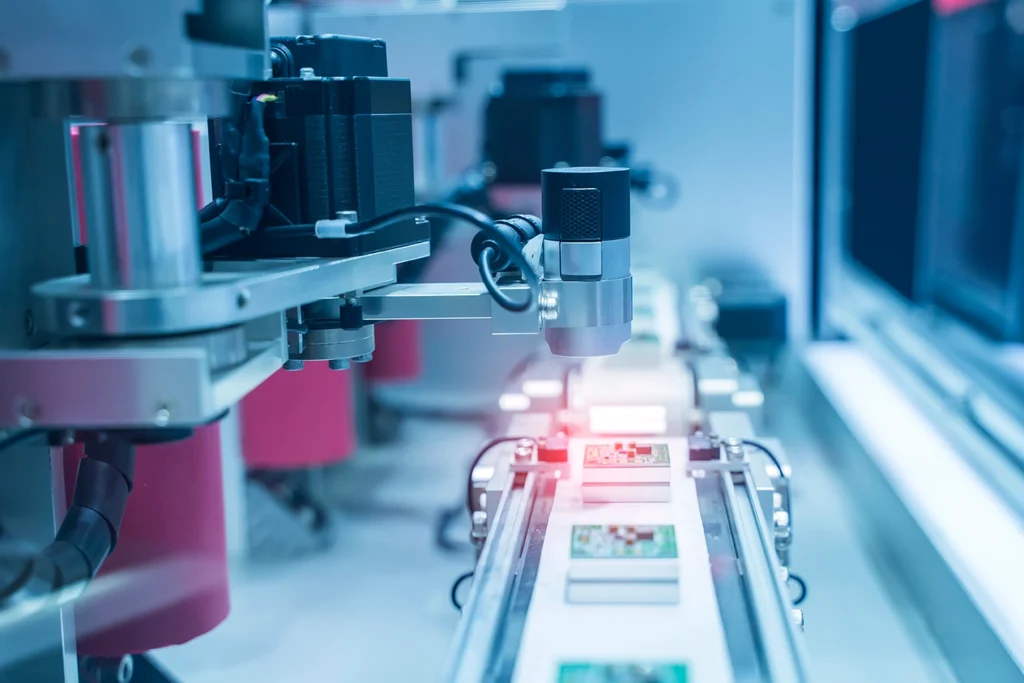
The integration of AI at the edge also addresses the growing need for data privacy and security. By processing data locally, sensitive information does not need to be transmitted to centralized cloud servers, reducing the risk of data breaches and ensuring compliance with data protection regulations. Moreover, edge AI reduces the bandwidth required for data transfer, as only the necessary information is sent to the cloud, optimizing network resources and reducing costs.
Training and Inference at the Edge
Training AI models involves feeding large datasets into algorithms to enable them to learn patterns and make predictions. Traditionally, this process requires significant computational power and is often performed in centralized data centers. However, advancements in edge computing and model optimization techniques have made it possible to train AI models at the edge.
One of the key techniques for enabling AI training at the edge is model optimization. This includes methods such as pruning, quantization, and low-rank adaptation, which reduce the size and complexity of AI models without compromising their performance. Pruning involves removing less important neurons or layers from a neural network, while quantization reduces the precision of the model’s weights, making it more efficient in terms of memory and computational requirements. Low-rank adaptation focuses on modifying only a subset of parameters, which is particularly useful for fine-tuning pre-trained models on specific tasks.
Inference, the process of making predictions using a trained AI model, is especially critical at the edge. It requires lower computational power compared to training and can be optimized for low-latency and energy-efficient operations. Edge devices equipped with AI inference capabilities can analyze data in real-time and provide immediate feedback. For example, in retail, edge AI can facilitate frictionless checkout experiences by instantly recognizing and processing items, while in smart cities, it can manage traffic and enhance public safety by analyzing real-time data from surveillance cameras and sensors.
The Role of Pluggables in the Network Edge
Optical transceivers are crucial in developing better AI systems by facilitating the rapid, reliable data transmission these systems need to do their jobs. High-speed, high-bandwidth connections are essential to interconnect data centers and supercomputers that host AI systems and allow them to analyze a massive volume of data.
In addition, optical transceivers are essential for facilitating the development of artificial intelligence-based edge computing, which entails relocating compute resources to the network’s periphery. This is essential for facilitating the quick processing of data from Internet-of-Things (IoT) devices like sensors and cameras, which helps minimize latency and increase reaction times.
Pluggables that fit this new AI era must be fast, smart, and adapt to multiple use cases and conditions. They will relay monitoring data back to the AI management layer in the central office. The AI management layer can then program transceiver interfaces from this telemetry data to change parameters and optimize the network.
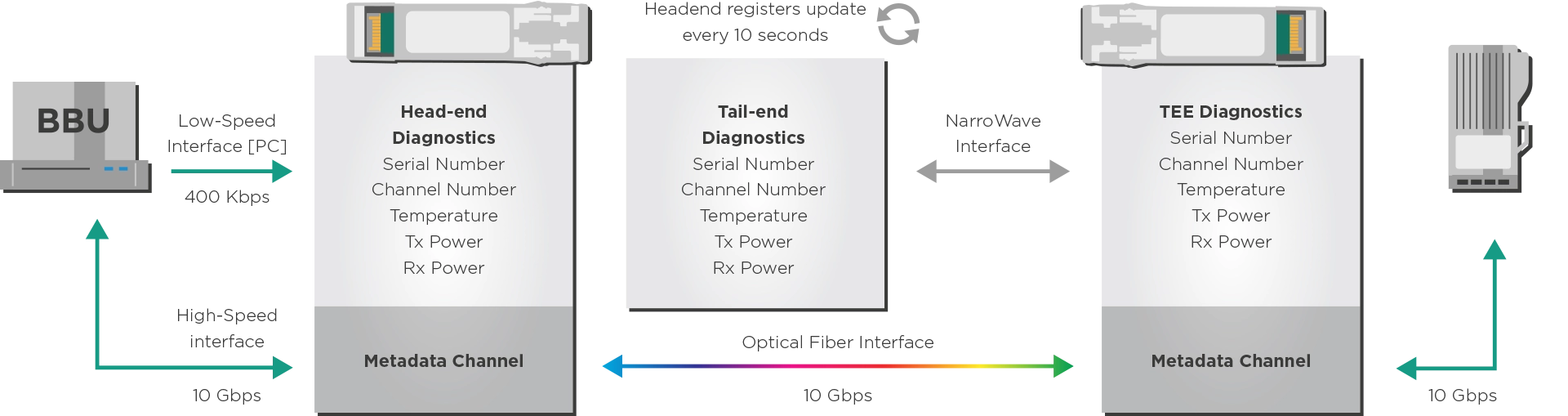
Takeaways
By bringing AI closer to the source of data generation, it enables real-time analytics, reduces latency, enhances data privacy, and optimizes network resources. Edge AI can foster innovation in areas such as autonomous vehicles, where real-time data processing is crucial for safe navigation and decision-making. In the healthcare sector, edge AI will enable more sophisticated patient monitoring systems, capable of diagnosing and responding to medical emergencies instantly. Moreover, edge AI will play a role in mobile networks, providing the necessary infrastructure to handle the massive amounts of data generated by connected devices.