AI and the New Drivers of Data Traffic
In this world, nothing can be said to be certain except death, taxes, and the growth of data traffic in communication networks. However, the causes of that growth vary over time depending on emerging technologies and shifting consumer behaviors.
The relationship between network capacity and data traffic closely mirrors the concept of induced demand in highway traffic management. Induced demand in the context of roadways refers to the phenomenon where increasing the number of lanes or expanding the road infrastructure to reduce congestion and accommodate more vehicles often leads to even higher traffic volumes. This is because the improved road capacity makes driving more appealing, thus encouraging more people to use their vehicles or to use them more often.
Similarly, as network capacity is increased—be it through the expansion of bandwidth or the introduction of more efficient data transmission—the network becomes capable of supporting higher loads and faster services. This improvement in network performance can encourage more data-intensive applications and services to be developed and used, such as high-definition video streaming, real-time gaming, and comprehensive Internet-of-things (IoT) solutions. As a result, the demand for data grows further, often at a pace that quickly meets or even exceeds the newly added capacity.
This article will tackle some recent key trends of the last couple of years that are driving the latest surge in data traffic.
5G and the Internet of Things
The Internet of Things (IoT) is a series of technologies interconnecting physical devices, allowing them to communicate, collect, and exchange data without human intervention. This connectivity enhances operational efficiency, improves safety, and reduces human labor in various environments—from industrial settings with automated production lines to everyday consumer use, such as smart home devices that enhance user convenience and energy efficiency.
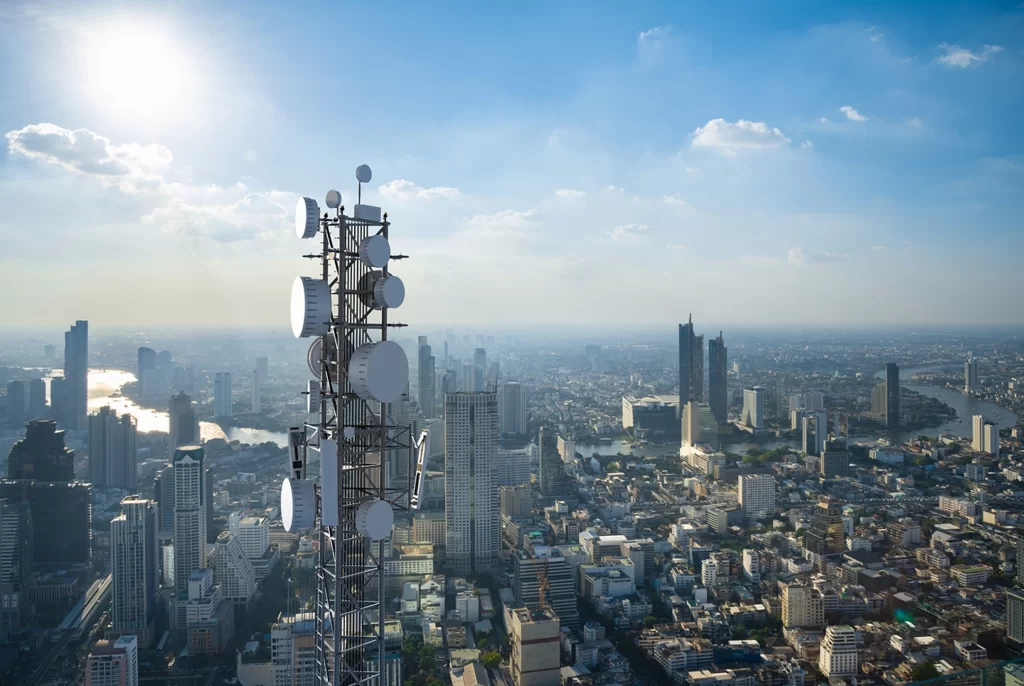
By converting ordinary objects into smart, connected components, IoT enables real-time data collection and analysis. This leads to more informed decision-making and predictive maintenance, which can significantly cut costs and increase productivity across multiple sectors.
The advent of 5G technology, with its promise of ultra-fast speeds and low latency, has enabled many IoT applications, turning mundane devices into smart, interconnected components of broader digital ecosystems. However, this means more devices contribute to the already vast data streams flowing through global networks.
Cloud Computing and the Edge
Cloud computing offers scalable and flexible IT resources over the Internet, allowing businesses to avoid the upfront cost and complexity of owning and maintaining their own IT infrastructure. By leveraging cloud services, organizations can access a wide array of computing resources on demand, such as servers, storage, databases, and software applications.
Meanwhile, applications such as IoT, AR/VR, and content delivery networks have driven the growth of edge computing. Edge computing complements cloud computing by processing data near the source rather than relying on a central data center. This is important for applications requiring real-time processing and low latency, such as autonomous vehicles, industrial automation, and smart city technologies. By minimizing the distance data must travel, edge computing reduces latency, increases data processing speed, and enhances sensitive data’s reliability and privacy.
As shown in Table 1, a data center on a town or suburb aggregation point could halve the latency compared to a centralized hyperscale data center. Enterprises with their own data center on-premises can reduce latencies by 12 to 30 times compared to hyperscale data centers.
Types of Edge Data Centres
Types of Edge | Data center | Location | Number of DCs per 10M people | Average Latency | Size | |
---|---|---|---|---|---|---|
On-premises edge | Enterprise site | Businesses | NA | 2-5 ms | 1 rack max | |
Network (Mobile) | Tower edge | Tower | Nationwide | 3000 | 10 ms | 2 racks max |
Outer edge | Aggregation points | Town | 150 | 30 ms | 2-6 racks | |
Inner edge | Core | Major city | 10 | 40 ms | 10+ racks | |
Regional edge | Regional edge | Regional | Major city | 100 | 50 ms | 100+ racks |
Not edge | Not edge | Hyperscale | State/national | 1 | 60+ ms | 5000+ racks |
As more people and organizations adopt cloud-based services and as these services become more data-intensive (e.g., high-definition video streaming, large-scale machine learning models), the volume of data traversing the internet continues to grow. While edge computing processes much of the data locally to reduce latency, it increases data traffic in the access networks connected to edge devices.
AI and Machine Learning
Artificial Intelligence (AI) and Machine Learning (ML) are deeply transforming many industries, enabling increased automation of man tasks. AI employs sophisticated algorithms to interpret data, automate decisions, and act upon those decisions. Machine learning, a branch of AI, focuses on algorithms that allow computers to learn from data to make predictions or decisions without explicit programming. This capability is essential for many applications, from spam detection in emails to more complex problems such as diagnosing diseases or managing traffic flows efficiently.
AI and ML significantly increase data traffic due to several factors:
- Data Collection: Training and operating AI/ML models require extensive data collection from varied sources. This data must be transmitted to where it can be processed, contributing to substantial network traffic.
- Connectivity Increase: Integrating AI in devices and services, such as IoT or smart devices, leads to more internet-connected devices and higher data volumes being transmitted to central servers for analysis.
- Complex Computations: AI and ML computations usually occur in cloud environments, necessitating high-capacity links for processing and subsequent results downloads.
The increasing complexity of AI processing will impact not just the interconnections between data centers but also the architectures inside the data center. AI nodes inside data center racks are normally connected via electrical or RF signals, while the racks are connected via optical fiber interconnects. However, as AI systems do more parallelized processing, data center racks run into electrical memory and power consumption constraints. These electrical interconnects between AI nodes are increasingly becoming a bottleneck in the ability of data center architectures to scale and handle the demands of AI models sustainably.
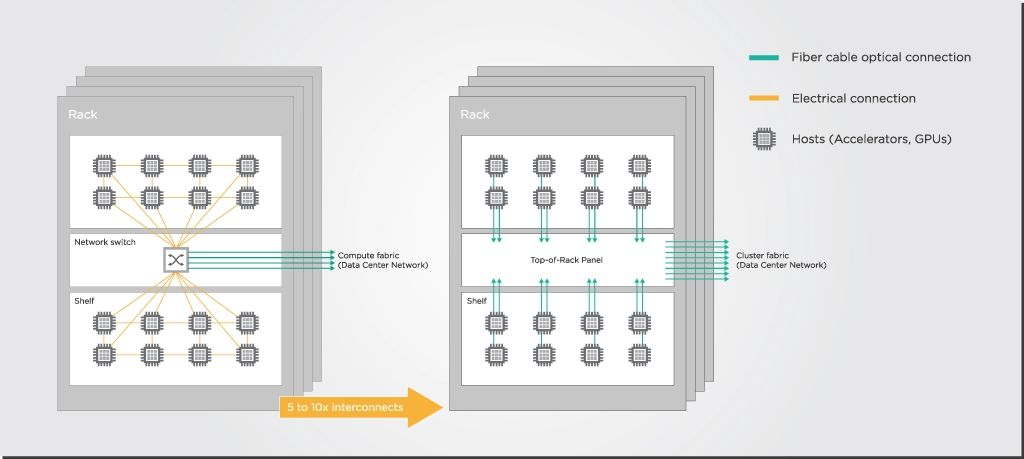
Takeaways
Emerging technologies such as 5G, IoT, cloud computing, and AI on data traffic are reshaping how data is generated, processed, and used across networks. Cloud computing continues democratizing access to technological resources, enabling businesses and individuals to leverage sophisticated tools without significant upfront investments. The Internet of Things (IoT) turns everyday objects into devices connected to 5G networks and the edge cloud. AI and machine learning represent perhaps the most significant drivers of increased data traffic, as they rely on massive data sets to train and operate.
These technologies will have a major impact on our society but will also need further innovations in network architecture to handle increased loads with minimal latency. Integrating AI across various devices and services not only multiplies the number of data-generating interactions but also complicates the data processing infrastructure, pushing the limits of current technologies and requiring new solutions to sustain growth. These are the challenges that drive the work of telecom and datacom companies all over the world.